AI in AR The same logic is driving the application of the RPA/AI combination to accounts receivable. AI algorithms are ideally suited to resolving some of the key prob- lems in receivables because they can learn from previous experience and behaviour patterns. This allows them to build up a picture of good and bad cred- its, early and late payers, fraudulent activity and even message repair and exception handling. One key receivables problem has always been the level of manual intervention required to cope with the mismatch between the way customers deal with billing and the way a particular treasury system would like them to. The multiple exceptions, errors and idiosyncrasies of the process defeat both the simplest sys- tems that used optical character recogni- tion (OCR) with templates and also more intelligent rule-based solutions. Now however, companies like US-based In the UK, Arria has developed natural across an international network and HighRadius, Receivable Savvy and Ger- language generation software (NLG) techniques such as natural language pro- many’s collectAI are using self-learning that is being used across a wide range of cessing and machine learning are being solutions to automate AR processes to industries to humanise and simplify the used to ‘understand’ the law, map com- make debt collection more efficient, analysis of data heavy reports. KPMG pliance needs and even analyse the costs reduce costs, improve cash flow and has been using innovations from of compliance. By treating regulations as increase customer retention rates. McLaren Applied Technologies (MAT) data, software will dynamically bring These types of software do everything in its audit processes where predictive compliance into the enterprise risk envi- from work out the best channel on which analytics automates evidence gathering ronment, enabling treasurers to take a to contact debtors to figuring out which and the production of complex data genuinely risk-based view of regulatory payments relate to which invoices despite reports, saving time and improving cli- compliance. customers’ habit of using one payment to ent services. Deloitte recently announced fully or partly pay multiple invoices and a partnership with Kira Systems to aid in These drivers have spawned an entire optimising early payment incentives to a contract and document reviewing, and RegTech industry that aims to replace particular customer using algorithmic has already rolled out a customised ver- the present combination of scattered invoice discounting to build intelligent sion for audit processes with further humans and fragmented legacy technol- supply chain finance solutions. applications being explored for tax and ogy to ensure that trades, customers and advisory practices. the company are compliant. Others, like YayPay look at a customer’s The pace of change in global regulation payment habits and behaviours and uses And J.P. Morgan’s Contract Intelligence machine learning to predict the potential (COIN) system has replaced the 360,000 and the complexity of AML/KYC and day of their payment. This forecast is hours spent each year by lawyers and OFAC sanctions compliance are too then used by the treasury team (or other loan officers interpreting commercial- much for existing instructional algo- automated solutions) to target the most loan agreements and other contracts. rithms and rules-based systems. The lat- significant outstanding debt. COIN runs on a cloud-based machine ter flag cash transactions over a certain learning system and as well as being currency amount, block transactions to AI in AP many times faster than its human coun- certain countries, use customer data to terparts it has also managed to help J.P. select accounts for additional monitor- In the same way, the advent of intelligent Morgan decrease the number of loan- ing, and categorise merchant accounts mobile bill processing technology able to servicing mistakes that are, in part, based on prior transactions. But they execute transactions to any schedule or set driven by the need to interpret 12,000 generate large numbers of false positives of rules, will transform accounts payable. new wholesale contracts every year. which need human intervention and they One set of solutions uses supervised Again, it is the sheer scale of the data cannot cope adequately with deliberate machine learning to teach a software tool crunching required that will force com- attempts at fraud or evasion. the key data points on a set of scanned panies to seek out this kind of solution. invoices. After the training phase, the AI- An AI solution ‘learns’ to identify prob- enabled tool can perform the data extrac- The next level lem transactions by analysing every data tion completely on its own and only brings point in the entire transaction database. invoices to the attention of a human where More complex compliance requires more It develops rules of its own based on, for it does not recognise them. sophisticated solutions. Even a medium- example, customer location, transaction sized firm may have to evaluate hun- timing, social media activity and rela- Here, the main benefit of artificial intel- dreds of tax and legal updates a week tionships with other customers. ligence is the ability to speed read huge www.eurofinance.com TREASURY PERSPECTIVES 2017/2018 // 11
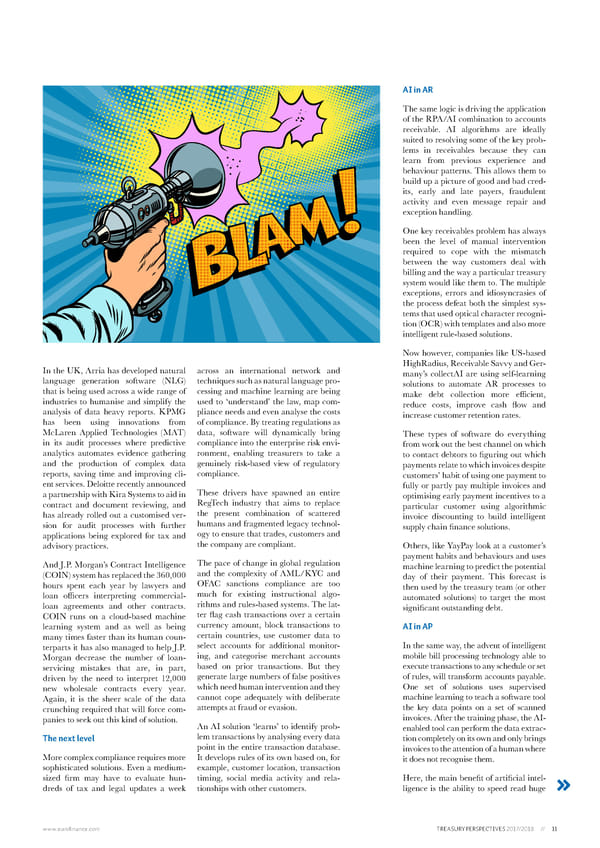